INVESTIGASI PERBANDINGAN COSINE SIMILARITY DAN EUCLIDEAN DISTANCE DALAM DETEKSI PHISHING ATTACK MENGGUNAKAN METODE K-NEAREST NEIGHBOR
DOI:
https://doi.org/10.21067/bimasakti.v6i2.10107Abstract
The development of information technology affects various aspects of life. However, this positive impact also opens up opportunities for growing cybercrime, known as cybercrime (Iman et al., 2020). These crimes, such as carding, hacking, and phishing, threaten security in the digital realm (Gulo et al., 2021). Phishing, as a form of cybercrime, involves sending fake links to steal victim information (Wibowo & Fatimah, 2017). In the midst of the development of information technology systems, data mining has emerged as a solution, enabling all valuable information from big data. K-Nearest Neighbors (KNN) is a machine learning algorithm used for classification and regression (Dewi Obert & Gusmana, 2018). In K-Nearest Neighbor, distance methods such as euclidean distance, Manhattan distance, cosine similarity, and jaccard similarity are commonly used. The focus of this research is on euclidean distance and cosine similarity which are considered efficient and commonly used. The evaluation results show that the second method, cosine similarity and Euclidean distance, has a similar level of accuracy and speed in detecting phishing attacks. However, Euclidean distance stands out in phishing detection with an accuracy rate of 87.70% and a speed of 0.0172. Meanwhile, cosine similarity reaches an accuracy rate of 87.57% with a speed of 0.0360. Looping analysis consistently confirms the Euclidean distance speed advantage. In phishing attack detection, Euclidean distance is proven to be more effective in accuracy and speed.
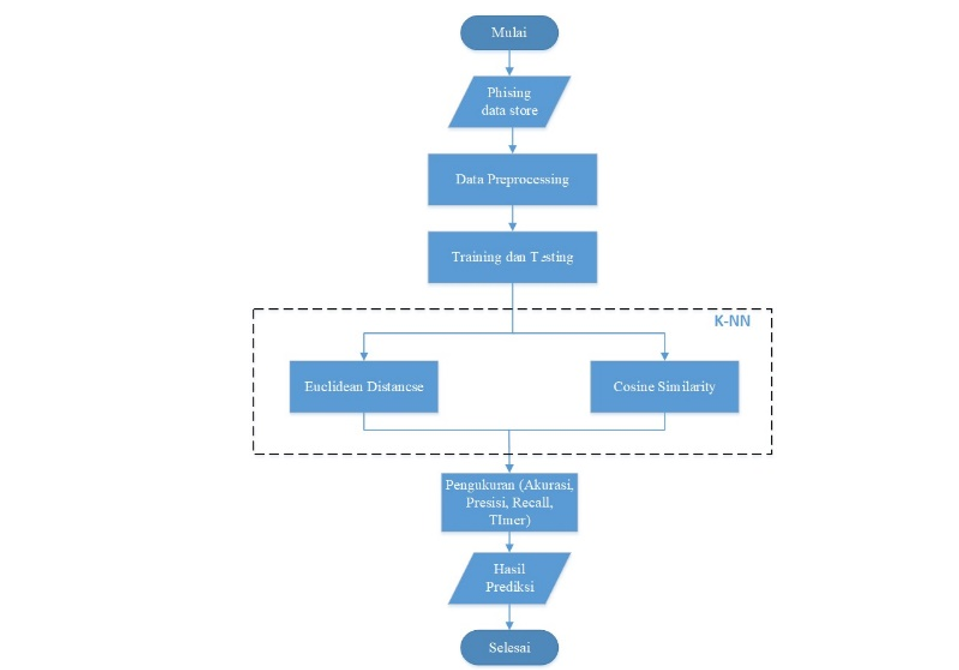